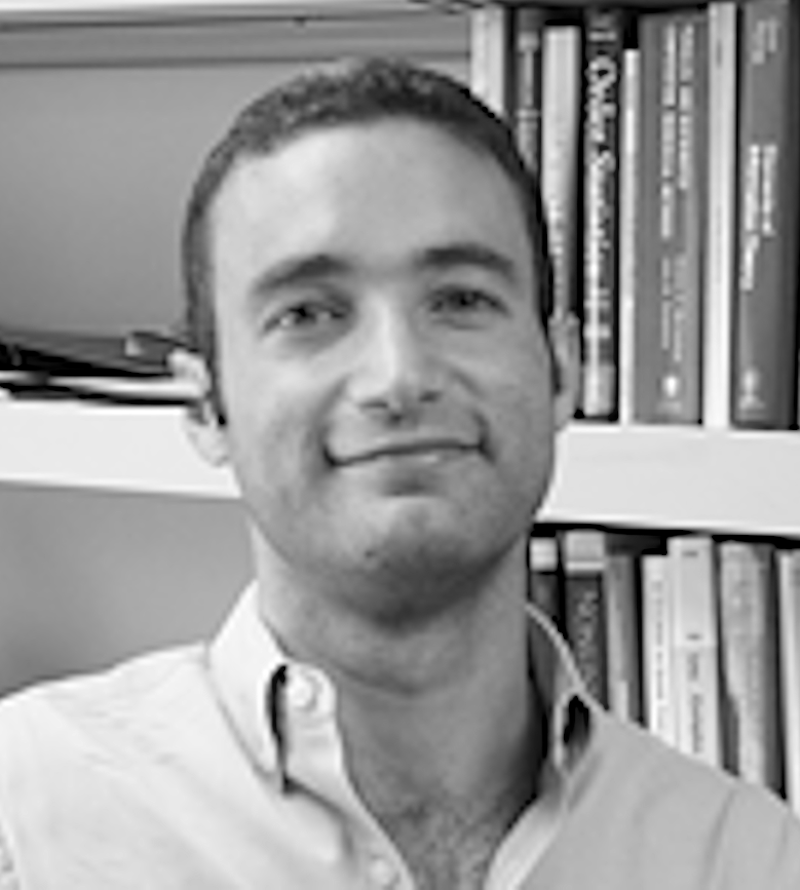
Osvaldo Simeone directs the King’s Communication, Learning, and Information Processing (KCLIP) lab and King’s College London, where he is a Professor of Information Engineering at the Department of Engineering. He received an M.Sc. degree (with honors) and a Ph.D. degree in information engineering from Politecnico di Milano, Milan, Italy, in 2001 and 2005, respectively. From 2006 to 2017, he was with the New Jersey Institute of Technology (NJIT). Dr Simeone is a co-recipient of the 2019 IEEE Communication Society Best Tutorial Paper Award, the 2018 IEEE Signal Processing Best Paper Award, the 2017 JCN Best Paper Award, the 2015 IEEE Communication Society Best Tutorial Paper Award and of the Best Paper Awards of IEEE SPAWC 2007 and IEEE WRECOM 2007.
From Learning to Meta-Learning for Reduced Training Overhead
Machine learning methods adapt the parameters of a model, constrained to lie in a given model class, by using a fixed learning procedure based on data or active observations. Adaptation is done on a per-task basis, and retraining is needed when the system configuration changes. Meta-learning provides a way to automatize the selection of an inductive bias, i.e., model class and/or learning procedure. With a meta-trained inductive bias, training of a machine learning model can be potentially carried out with reduced training data and/or time complexity. This talk provides a high-level introduction to meta-learning with applications to communication systems.
Hits: 434